AIRobers
Ph.D. Students
M.Sc. Students
Alumni
Current Members
Dingyi Sun (Ph.D. Student)
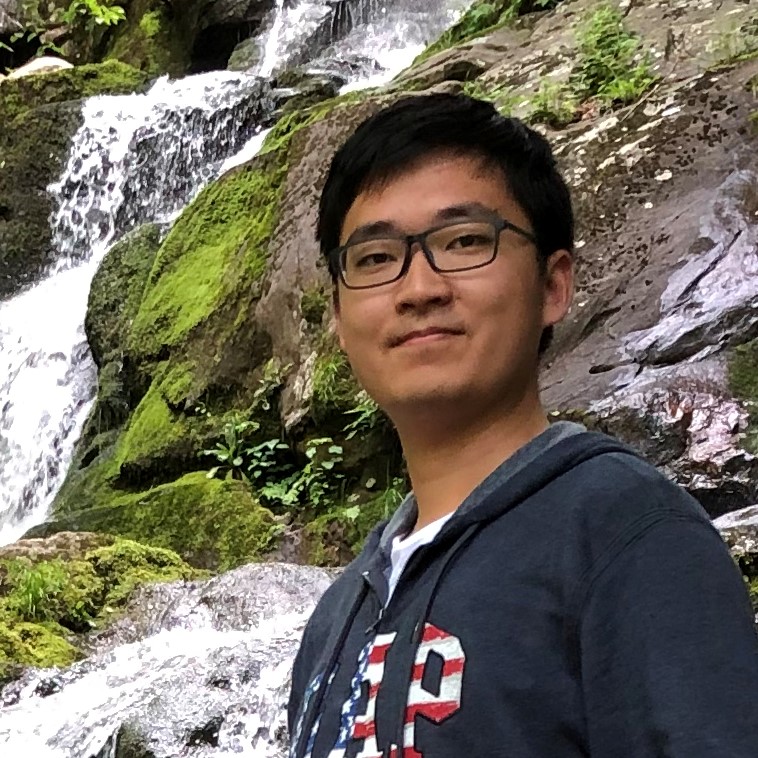
Dingyi received a B.Eng. in Electrical Engineering and Automation from Huazhong University of Science and Technology in 2018 and an M.S. in Electrical and Computer Engineering (Robotics Track) from the University of Michigan in 2020. He is interested in path planning, machine learning, and robotic perception.
Jiaqi Tan (Ph.D. Student)
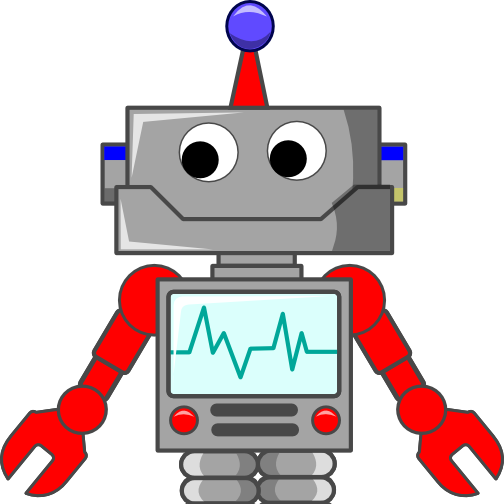
Jiaqi received a B.Eng. in Computer Science and Technology from Shenzhen University in 2019 and an M.Sc. in Computing Science from Simon Fraser University in 2022.
-
This paper presents a vector HD-mapping algorithm that formulates the mapping as a tracking task and uses a history of memory latents to ensure consistent reconstructions over time. Our method, MapTracker, accumulates a sensor stream into memory buffers of two latent representations: 1) Raster latents in the bird’s-eye-view (BEV) space and 2) Vector latents over the road elements (i.e., pedestrian-crossings, lane-dividers, and road-boundaries). The approach borrows the query propagation paradigm from the tracking literature that explicitly associates tracked road elements from the previous frame to the current, while fusing a subset of memory latents selected with distance strides to further enhance temporal consistency. A vector latent is decoded to reconstruct the geometry of a road element. The paper further makes benchmark contributions by 1) Improving processing code for existing datasets to produce consistent ground truth with temporal alignments and 2) Augmenting existing mAP metrics with consistency checks. MapTracker significantly outperforms existing methods on both nuScenes and Agroverse2 datasets by over 8% and 19% on the conventional and the new consistency-aware metrics, respectively. The code will be available on our project page: https://map-tracker.github.io.
@inproceedings{ChenECCV24, author = {Jiacheng Chen and Yuefan Wu and Jiaqi Tan and Hang Ma and Yasutaka Furukawa}, booktitle = {European Conference on Computer Vision}, pages = {(in print)}, title = {MapTracker: Tracking with Strided Memory Fusion for Consistent Vector HD Mapping}, year = {2024} }
Jingtao Tang (Ph.D. Student)
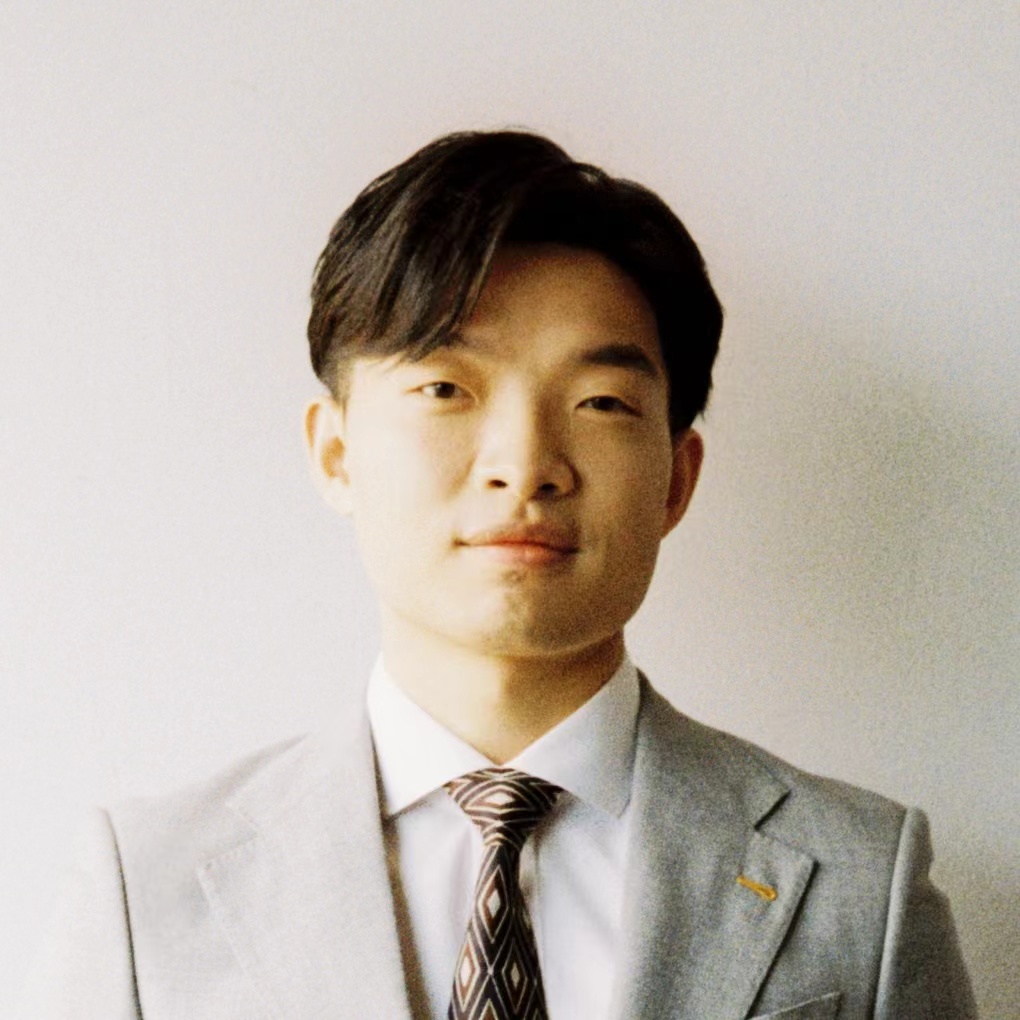
Jingtao received a B.Eng. and an M.S., both in Software Engineering from East China Normal University, in 2018 and 2021, respectively. His research interests include planning and scheduling, multi-agent systems, combinatorial optimization, and machine learning. More information can be found on his homepage.
-
We introduce the Multi-Robot Connected Fermat Spiral (MCFS), a novel algorithmic framework for Multi-Robot Coverage Path Planning (MCPP) that adapts Connected Fermat Spiral (CFS) from the computer graphics community to multi-robot coordination for the first time. MCFS uniquely enables the orchestration of multiple robots to generate coverage paths that contour around arbitrarily shaped obstacles, a feature that is notably lacking in traditional methods. Our framework not only enhances area coverage and optimizes task performance, particularly in terms of makespan, for workspaces rich in irregular obstacles but also addresses the challenges of path continuity and curvature critical for non-holonomic robots by generating smooth paths without decomposing the workspace. MCFS solves MCPP by constructing a graph of isolines and transforming MCPP into a combinatorial optimization problem, aiming to minimize the makespan while covering all vertices. Our contributions include developing a unified CFS version for scalable and adaptable MCPP, extending it to MCPP with novel optimization techniques for cost reduction and path continuity and smoothness, and demonstrating through extensive experiments that MCFS outperforms existing MCPP methods in makespan, path curvature, coverage ratio, and overlapping ratio. Our research marks a significant step in MCPP, showcasing the fusion of computer graphics and automated planning principles to advance the capabilities of multi-robot systems in complex environments. Our code is publicly available at https://github.com/reso1/MCFS.
@inproceedings{TangICAPS24, author = {Jingtao Tang and Hang Ma}, booktitle = {International Conference on Automated Planning and Scheduling}, pages = {579--587}, title = {Multi-Robot Connected Fermat Spiral Coverage}, year = {2024} }
-
We study graph-based Multi-Robot Coverage Path Planning (MCPP) that aims to compute coverage paths for multiple robots to cover all vertices of a given 2D grid terrain graph $G$. Existing graph-based MCPP algorithms first compute a tree cover on $G$---a forest of multiple trees that cover all vertices---and then employ the Spanning Tree Coverage (STC) paradigm to generate coverage paths on the decomposed graph $D$ of the terrain graph $G$ by circumnavigating the edges of the computed trees, aiming to optimize the makespan (i.e., the maximum coverage path cost among all robots). In this paper, we take a different approach by exploring how to systematically search for good coverage paths directly on $D$. We introduce a new algorithmic framework, called LS-MCPP, which leverages a local search to operate directly on $D$. We propose a novel standalone paradigm, Extended-STC (ESTC), that extends STC to achieve complete coverage for MCPP on any decomposed graphs, even those resulting from incomplete terrain graphs. Furthermore, we demonstrate how to integrate ESTC with three novel types of neighborhood operators into our framework to effectively guide its search process. Our extensive experiments demonstrate the effectiveness of LS-MCPP, consistently improving the initial solution returned by two state-of-the-art baseline algorithms that compute suboptimal tree covers on $G$, with a notable reduction in makespan by up to 35.7% and 30.3%, respectively. Moreover, LS-MCPP consistently matches or surpasses the results of optimal tree cover computation, achieving these outcomes with orders of magnitude faster runtime, thereby showcasing its significant benefits for large-scale real-world coverage tasks.
@inproceedings{TangAAAI24, author = {Jingtao Tang and Hang Ma}, booktitle = {{AAAI} Conference on Artificial Intelligence}, pages = {17567--17574}, title = {Large-Scale Multi-Robot Coverage Path Planning via Local Search}, year = {2024} }
-
We investigate time-optimal Multi-Robot Coverage Path Planning (MCPP) for both unweighted and weighted terrains, which aims to minimize the coverage time, defined as the maximum travel time of all robots. Specifically, we focus on a reduction from MCPP to Min-Max Rooted Tree Cover (MMRTC). For the first time, we propose a Mixed Integer Programming (MIP) model to optimally solve MMRTC, resulting in an MCPP solution with a coverage time that is provably at most four times the optimal. Moreover, we propose two suboptimal yet effective heuristics that reduce the number of variables in the MIP model, thus improving its efficiency for large-scale MCPP instances. We show that both heuristics result in reduced-size MIP models that remain complete (i.e., guaranteed to find a solution if one exists) for all MMRTC instances. Additionally, we explore the use of model optimization warm-startup to further improve the efficiency of both the original MIP model and the reduced-size MIP models. We validate the effectiveness of our MIP-based MCPP planner through experiments that compare it with two state-of-the-art MCPP planners on various instances, demonstrating a reduction in the coverage time by an average of $27.65%$ and $23.24%$ over them, respectively.
@article{TangRAL23, author = {Jingtao Tang and Hang Ma}, journal = {IEEE Robotics and Automation Letters}, number = {10}, pages = {6491--6498}, title = {Mixed Integer Programming for Time-Optimal Multi-Robot Coverage Path Planning with Efficient Heuristics}, volume = {8}, year = {2023} }
Yifan Yang (Ph.D. Student)
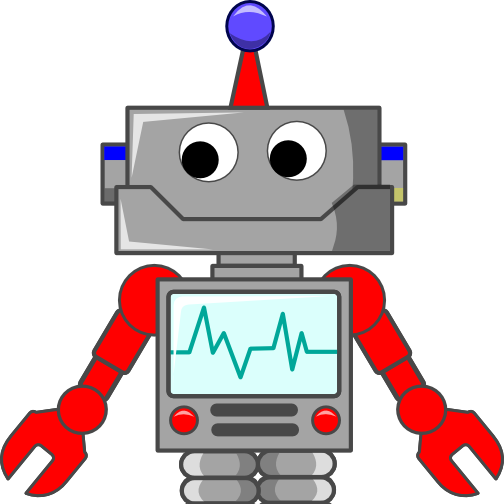
Yifan received a B.Eng. and an M.Eng., both in Computer Science and Technology from Harbin Institute of Technology, in 2020 and 2024, respectively.
Zining Mao (M.Sc. Student)
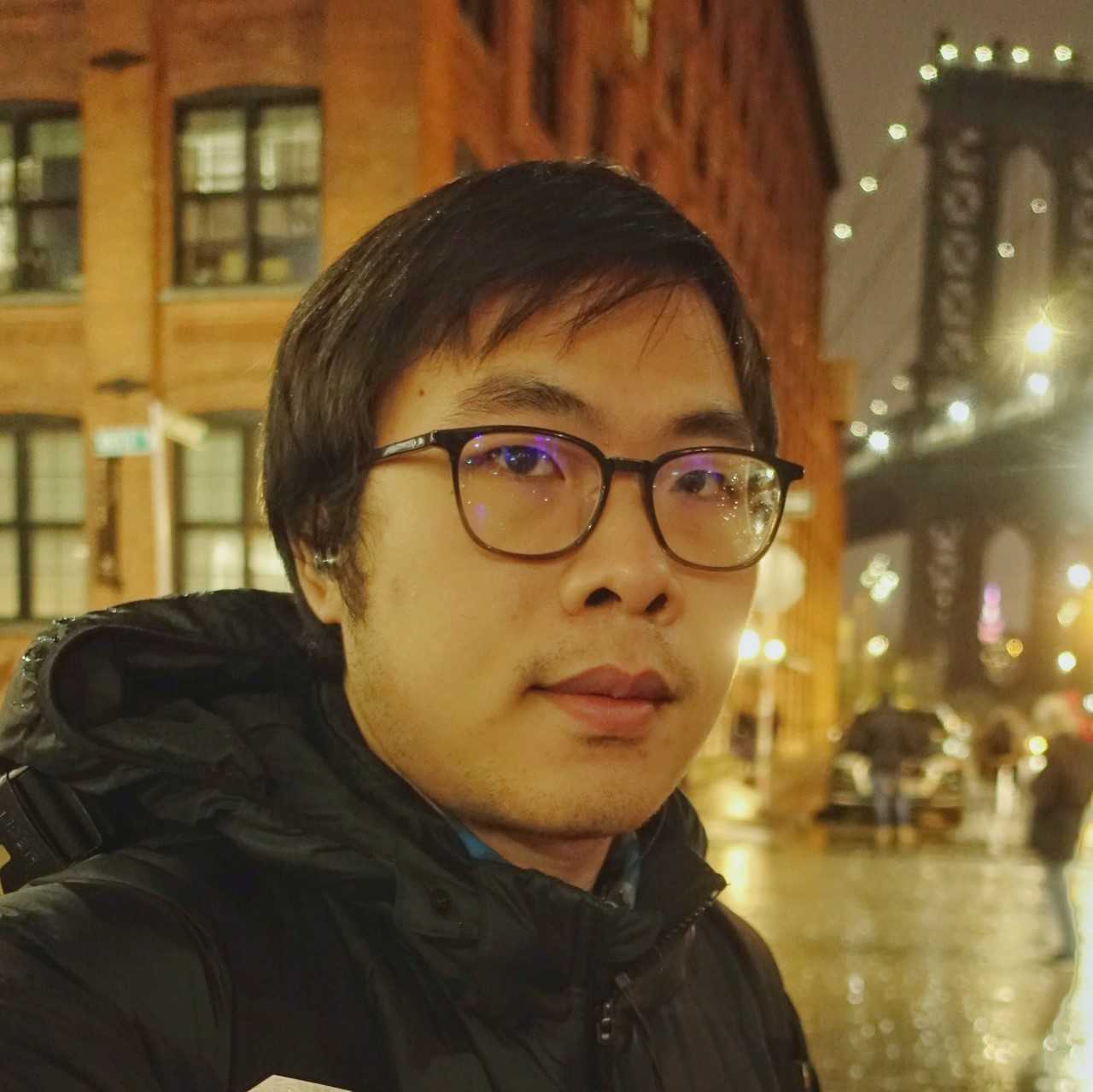
Zining received a B.Sc. in Computer Science from New York University Shanghai in 2021. He is interested in reinforcement learning and multi-agent systems.
Ervin Samuel (M.Sc. Student)
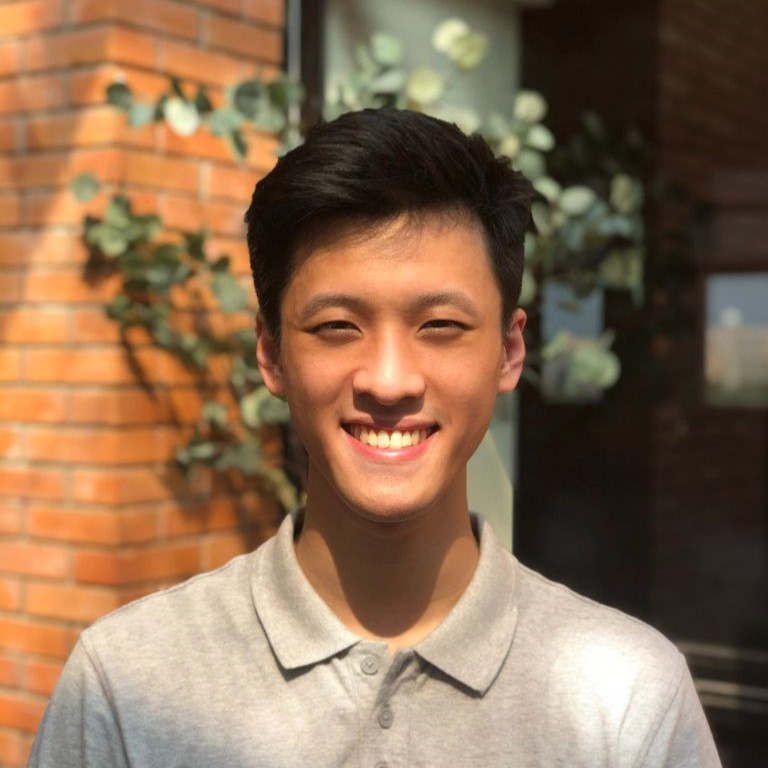
Ervin received a B.Sc. in Computer Science from National Tsing Hua University in 2022. He is interested in artificial intelligence, particularly reinforcement learning and its application to path planning.
Alumni
Danoosh Chamani (Former M.Sc. Student)
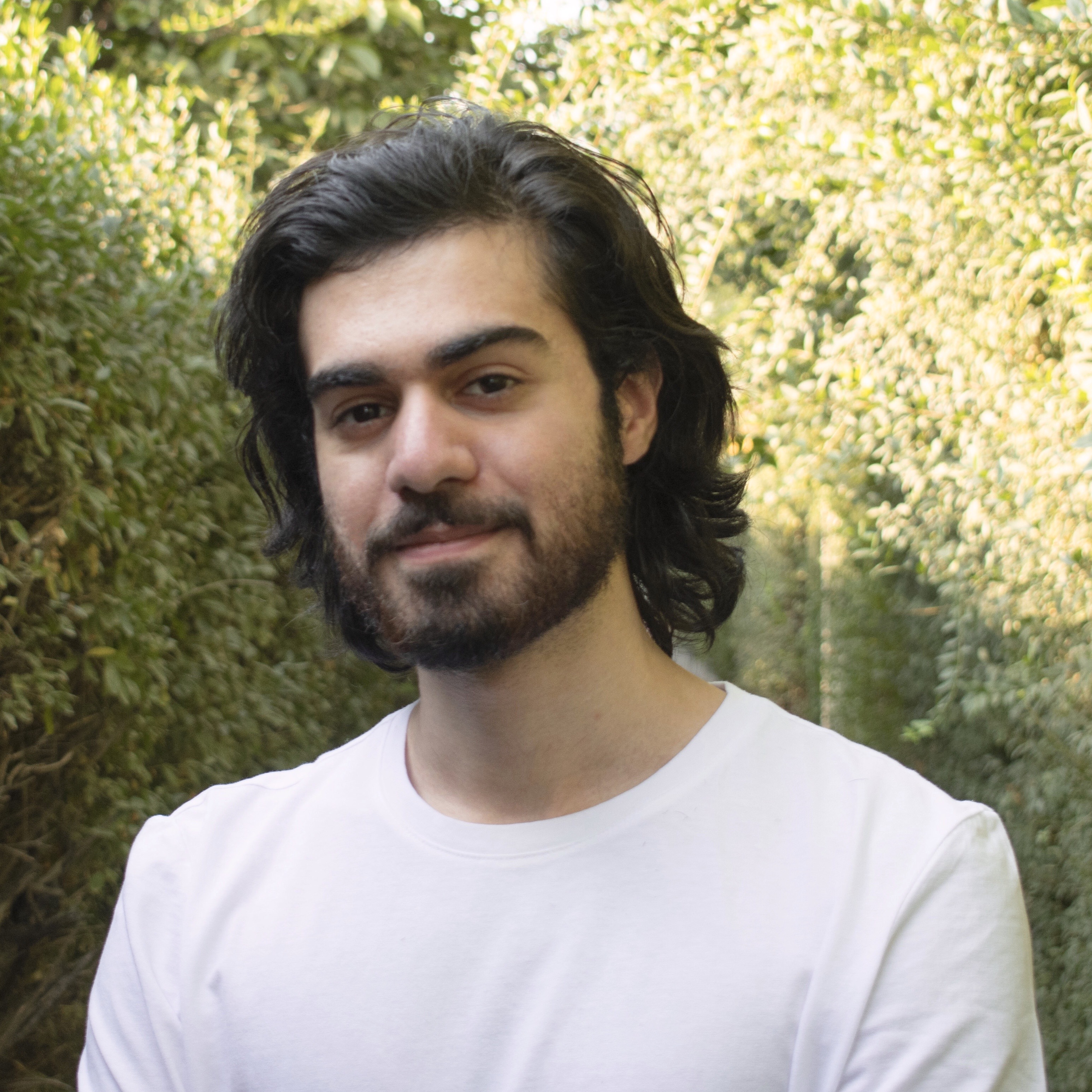
Danoosh received a B.Sc. in Computer Software Engineering from the University of Tehran in 2019 and an M.Sc. in Computing Science from Simon Fraser University in 2022. He is interested in reinforcement learning, machine learning, and robotics.
Last seen: Data Scientist at Health Canada
Baiyu Li (Former M.Sc. Student)
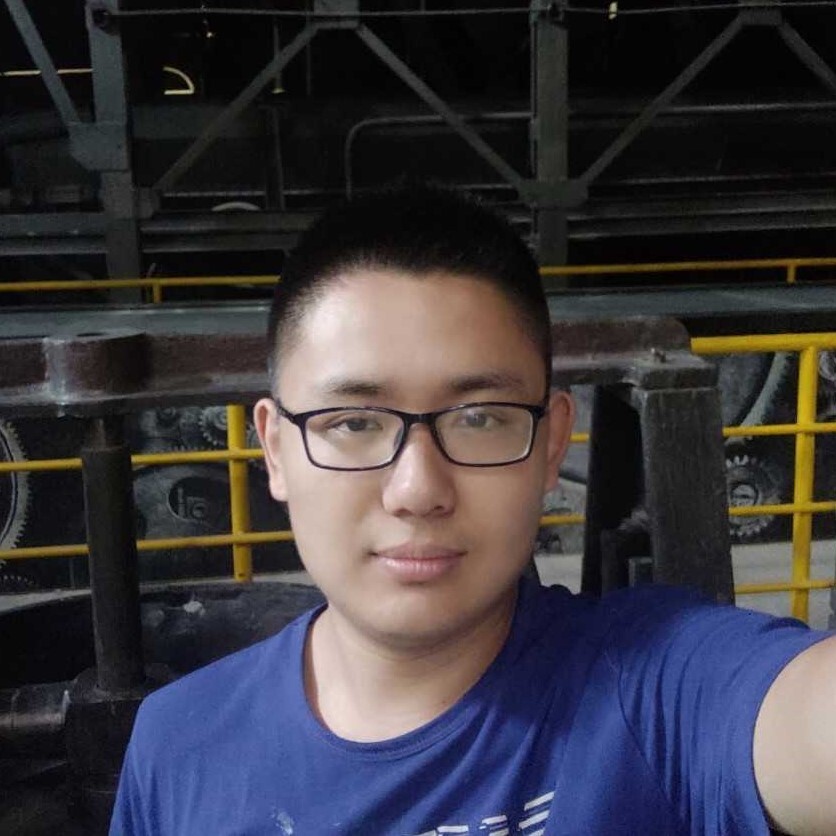
Baiyu received a B.Eng. in Computer Science from Northeastern University (Shenyang, China) in 2020 and an M.Sc. in Computing Science from Simon Fraser University in 2023. He is interested in path planning, multi-agent system, and parallel computing.
Last seen: Software Engineer at TikTok
Previously seen: Software Developer at Fortinet
Previously seen: Software Developer at Fortinet
-
We introduce a new problem formulation, Double-Deck Multi-Agent Pickup and Delivery (DD-MAPD), which models the multi-robot shelf rearrangement problem in automated warehouses. DD-MAPD extends both Multi-Agent Pickup and Delivery (MAPD) and Multi-Agent Path Finding (MAPF) by allowing agents to move beneath shelves or lift and deliver a shelf to an arbitrary location, thereby changing the warehouse layout. We show that solving DD-MAPD is NP-hard. To tackle DD-MAPD, we propose MAPF-DECOMP, an algorithmic framework that decomposes a DD-MAPD instance into a MAPF instance for coordinating shelf trajectories and a subsequent MAPD instance with task dependencies for computing paths for agents. We also present an optimization technique to improve the performance of MAPF-DECOMP and demonstrate how to make MAPF-DECOMP complete for well-formed DD-MAPD instances, a realistic subclass of DD-MAPD instances. Our experimental results demonstrate the efficiency and effectiveness of MAPF-DECOMP, with the ability to compute high-quality solutions for large-scale instances with over one thousand shelves and hundreds of agents in just minutes of runtime.
@article{LiRAL23, author = {Baiyu Li and Hang Ma}, journal = {IEEE Robotics and Automation Letters}, number = {6}, pages = {3701--3708}, title = {Double-Deck Multi-Agent Pickup and Delivery: Multi-Robot Rearrangement in Large-Scale Warehouses}, volume = {8}, year = {2023} }
Qiushi Lin (Former M.Sc. Student)
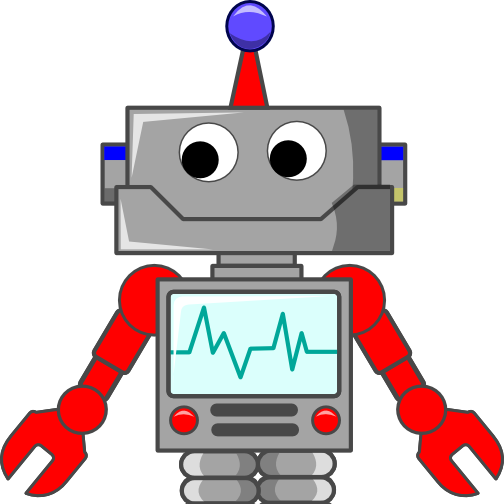
Qiushi received a B.Eng. in Computer Science and Technology from Southern University of Science and Technology in 2020 and an M.Sc. in Computing Science from Simon Fraser University in 2023. He is interested in machine learning, reinforcement learning, and multi-agent system. More information can be found on his homepage.
Last seen: Ph.D. Student at Simon Fraser University
-
We study a decentralized version of Moving Agents in Formation (MAiF), a variant of Multi-Agent Path Finding aiming to plan collision-free paths for multiple agents with the dual objectives of reaching their goals quickly while maintaining a desired formation. The agents must balance these objectives under conditions of partial observation and limited communication. The formation maintenance depends on the joint state of all agents, whose dimensionality increases exponentially with the number of agents, rendering the learning process intractable. Additionally, learning a single policy that can accommodate different linear preferences for these two objectives presents a significant challenge. In this paper, we propose Mean-Field Control with Envelop Q-learning (MFC-EQ), a scalable and adaptable learning framework for this bi-objective multi-agent problem. We approximate the dynamics of all agents using mean-field theory while learning a universal preference-agnostic policy through envelop Q-learning. Our empirical evaluation of MFC-EQ across numerous instances shows that it outperforms state-of-the-art centralized MAiF baselines. Furthermore, MFC-EQ effectively handles more complex scenarios where the desired formation changes dynamically -- a challenge that existing MAiF planners cannot address.
@inproceedings{LinIROS24, author = {Qiushi Lin and Hang Ma}, booktitle = {IEEE/RSJ International Conference on Intelligent Robots and Systems}, pages = {(in print)}, title = {MFC-EQ: Mean-Field Control with Envelope Q-Learning for Moving Decentralized Agents in Formation}, year = {2024} }
-
Multi-Agent Path Finding (MAPF) is a crucial component for many large-scale robotic systems, where agents must plan their collision-free paths to their given goal positions. Recently, multi-agent reinforcement learning has been introduced to solve the partially observable variant of MAPF by learning a decentralized single-agent policy in a centralized fashion based on each agent's partial observation. However, existing learning-based methods are ineffective in achieving complex multi-agent cooperation, especially in congested environments, due to the non-stationarity of this setting. To tackle this challenge, we propose a multi-agent actor-critic method called Soft Actor-Critic with Heuristic-Based Attention (SACHA), which employs novel heuristic-based attention mechanisms for both the actors and critics to encourage cooperation among agents. SACHA learns a neural network for each agent to selectively pay attention to the shortest path heuristic guidance from multiple agents within its field of view, thereby allowing for more scalable learning of cooperation. SACHA also extends the existing multi-agent actor-critic framework by introducing a novel critic centered on each agent to approximate $Q$-values. Compared to existing methods that use a fully observable critic, our agent-centered multi-agent actor-critic method results in more impartial credit assignment and better generalizability of the learned policy to MAPF instances with varying numbers of agents and types of environments. We also implement SACHA(C), which embeds a communication module in the agent's policy network to enable information exchange among agents. We evaluate both SACHA and SACHA(C) on a variety of MAPF instances and demonstrate decent improvements over several state-of-the-art learning-based MAPF methods with respect to success rate and solution quality.
@article{LinRAL23, author = {Qiushi Lin and Hang Ma}, journal = {IEEE Robotics and Automation Letters}, number = {8}, pages = {2377--3766}, title = {SACHA: Soft Actor-Critic with Heuristic-Based Attention for Partially Observable Multi-Agent Path Finding}, volume = {8}, year = {2023} }
Qinghong Xu (Former M.Sc. Student)
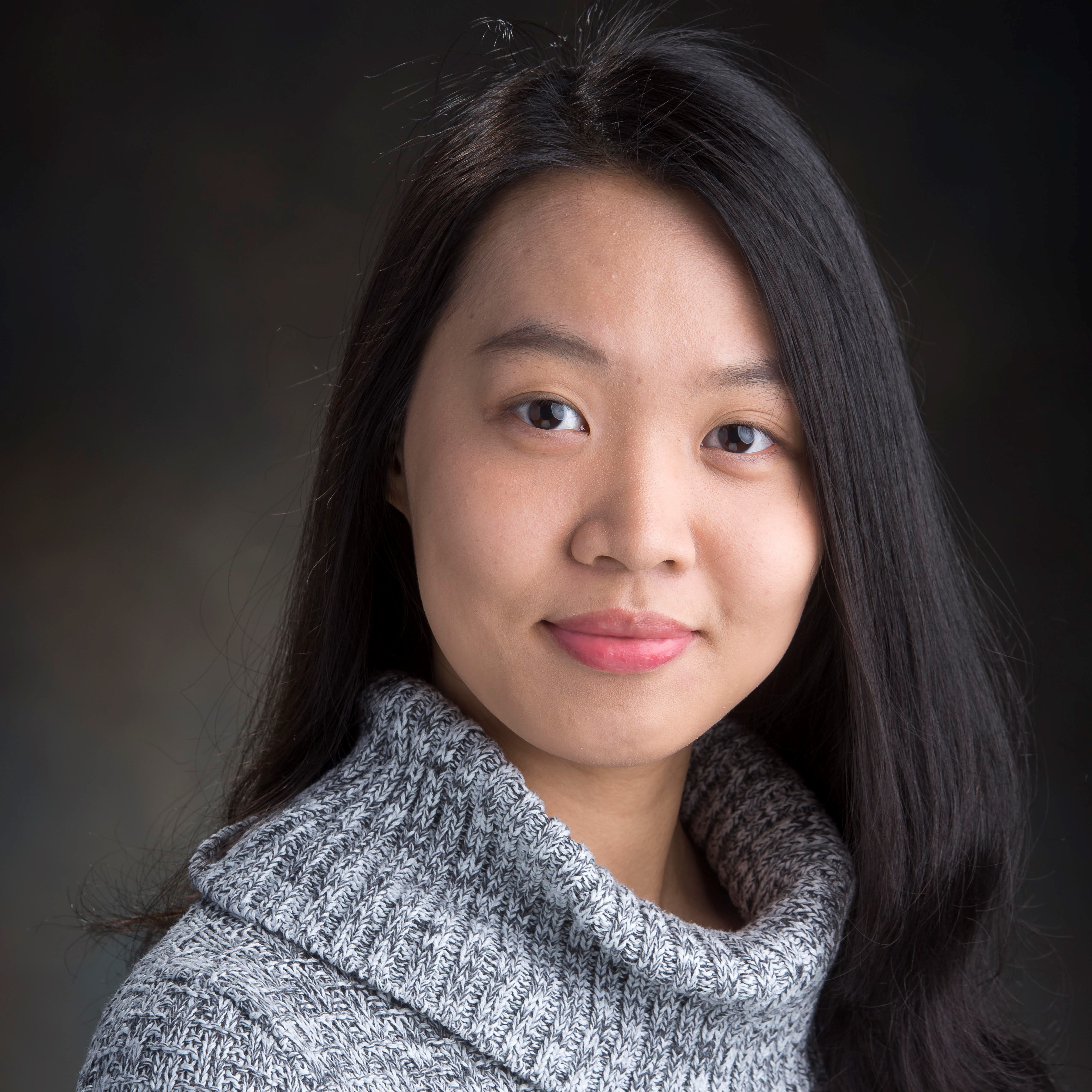
Qinghong received a B.S. in Computational Mathematics from Xiamen University in 2017 and an M.S. in Computational and Applied Mathematics in 2019 and an M.Sc. in Computing Science in 2022, both from Simon Fraser University. She is interested in multi-agent systems, path planning, and machine learning.
Last seen: Software Development Engineer at Amazon
-
In this work, we consider the Multi-Agent Pickup-and-Delivery (MAPD) problem, where agents constantly engage with new tasks and need to plan collision-free paths to execute them. To execute a task, an agent needs to visit a pair of goal locations, consisting of a pickup location and a delivery location. We propose two variants of an algorithm that assigns a sequence of tasks to each agent using the anytime algorithm Large Neighborhood Search (LNS) and plans paths using the Multi-Agent Path Finding (MAPF) algorithm Priority-Based Search (PBS). LNS-PBS is complete for well-formed MAPD instances, a realistic subclass of MAPD instances, and empirically more effective than the existing complete MAPD algorithm CENTRAL. LNS-wPBS provides no completeness guarantee but is empirically more efficient and stable than LNS-PBS. It scales to thousands of agents and thousands of tasks in a large warehouse and is empirically more effective than the existing scalable MAPD algorithm HBH+MLA*. LNS-PBS and LNS-wPBS also apply to a more general variant of MAPD, namely the Multi-Goal MAPD (MG-MAPD) problem, where tasks can have different numbers of goal locations.
@inproceedings{XuIROS22, author = {Qinghong Xu and Jiaoyang Li and Sven Koenig and Hang Ma}, booktitle = {{IEEE/RSJ} International Conference on Intelligent Robots and System}, pages = {9964--9971}, title = {Multi-Goal Multi-Agent Pickup and Delivery}, year = {2022} }
Xinyi Zhong (Former M.Sc. Student)
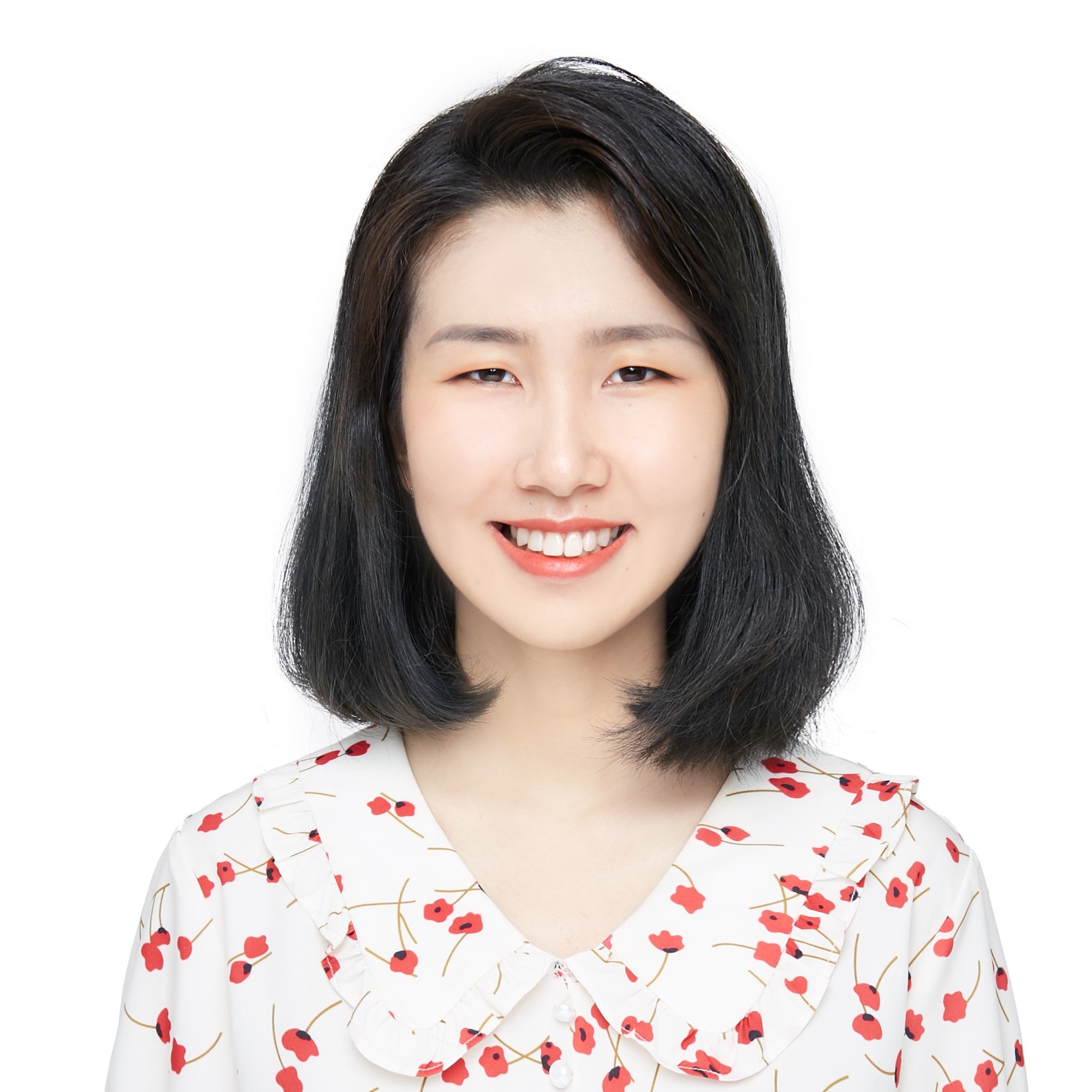
Xinyi received a B.C.S. Honours in Computer Science from Carleton University in 2019 and an M.Sc. in Computing Science from Simon Fraser University in 2021. She is interested in path planning, multi-agent system, and robotics.
Last seen: Software Development Engineer at Amazon
-
We formalize and study the multi-goal task assignment and pathfinding (MG-TAPF) problem from theoretical and algorithmic perspectives. The MG-TAPF problem is to compute an assignment of tasks to agents, where each task consists of a sequence of goal locations, and collision-free paths for the agents that visit all goal locations of their assigned tasks in sequence. Theoretically, we prove that the MG-TAPF problem is NP-hard to solve optimally. We present algorithms that build upon algorithmic techniques for the multi-agent pathfinding problem and solve the MG-TAPF problem optimally and bounded-suboptimally. We experimentally compare these algorithms on a variety of different benchmark domains.
@inproceedings{ZhongICRA22, author = {Xinyi Zhong and Jiaoyang Li and Sven Koenig and Hang Ma}, booktitle = {IEEE International Conference on Robotics and Automation}, pages = {10731--10737}, title = {Optimal and Bounded-Suboptimal Multi-Goal Task Assignment and Path Finding}, year = {2022} }
Ziyuan Ma (Former Undergraduate Student)
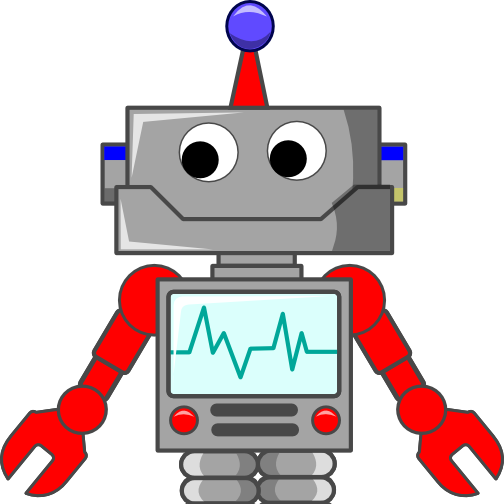
Ziyuan received a B.Sc. in Computing Science from Simon Fraser University in 2020. He was an undergraduate research student in our lab in 2020/2021.
-
Multi-Agent Path Finding (MAPF) is essential to large-scale robotic systems. Recent methods have applied reinforcement learning (RL) to learn decentralized polices in partially observable environments. A fundamental challenge of obtaining collision-free policy is that agents need to learn cooperation to handle congested situations. This paper combines communication with deep Q-learning to provide a novel learning based method for MAPF, where agents achieve cooperation via graph convolution. To guide RL algorithm on long-horizon goal-oriented tasks, we embed the potential choices of shortest paths from single source as heuristic guidance instead of using a specific path as in most existing works. Our method treats each agent independently and trains the model from a single agent’s perspective. The final trained policy is applied to each agent for decentralized execution. The whole system is distributed during training and is trained under a curriculum learning strategy. Empirical evaluation in obstacle-rich environment indicates the high success rate with low average step of our method.
@inproceedings{MaICRA21, author = {Ziyuan Ma and Yudong Luo and Hang Ma}, booktitle = {IEEE International Conference on Robotics and Automation}, pages = {8699--8705}, title = {Distributed Heuristic Multi-Agent Path Finding with Communication}, year = {2021} }
Yudong Luo (Former Visitor)
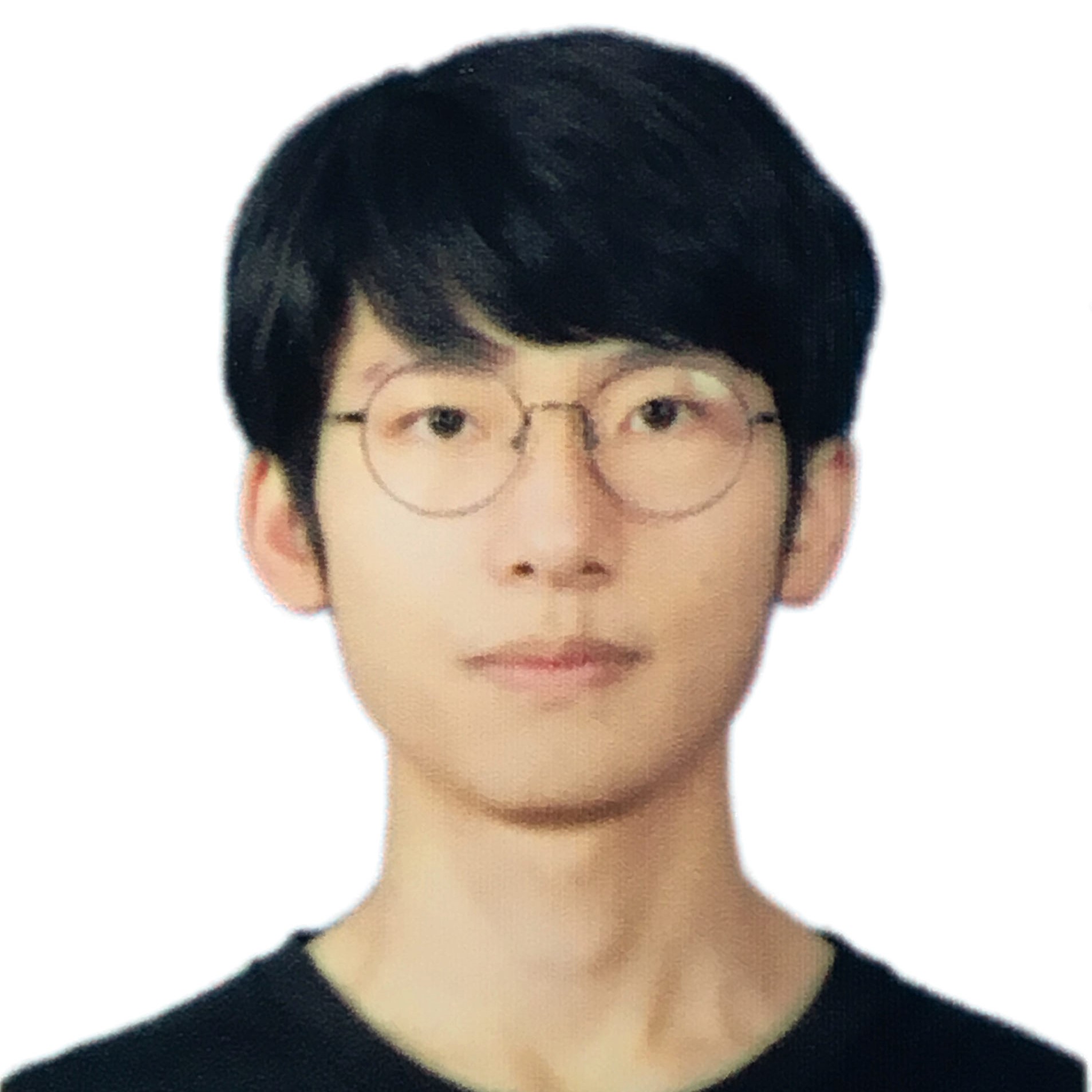
Yudong is a Ph.D. student at the University of Waterloo. He received a B.Eng. in Computer Science from Shanghai Jiao Tong University in 2018 and an M.Sc. in Computing Science from Simon Fraser University in 2020. He is interested in reinforcement learning, machine learning, and multi-agent system. Yudong visited our lab for 12 months in 2020/2021. More information can be found on his homepage.
-
Multi-Agent Path Finding (MAPF) is essential to large-scale robotic systems. Recent methods have applied reinforcement learning (RL) to learn decentralized polices in partially observable environments. A fundamental challenge of obtaining collision-free policy is that agents need to learn cooperation to handle congested situations. This paper combines communication with deep Q-learning to provide a novel learning based method for MAPF, where agents achieve cooperation via graph convolution. To guide RL algorithm on long-horizon goal-oriented tasks, we embed the potential choices of shortest paths from single source as heuristic guidance instead of using a specific path as in most existing works. Our method treats each agent independently and trains the model from a single agent’s perspective. The final trained policy is applied to each agent for decentralized execution. The whole system is distributed during training and is trained under a curriculum learning strategy. Empirical evaluation in obstacle-rich environment indicates the high success rate with low average step of our method.
@inproceedings{MaICRA21, author = {Ziyuan Ma and Yudong Luo and Hang Ma}, booktitle = {IEEE International Conference on Robotics and Automation}, pages = {8699--8705}, title = {Distributed Heuristic Multi-Agent Path Finding with Communication}, year = {2021} }